本文已被:浏览 1282次 下载 2323次
投稿时间:2018-08-22 修订日期:2019-11-05
投稿时间:2018-08-22 修订日期:2019-11-05
中文摘要: 基于TIGGE资料中的ECMWF、UKMO、JMA、CMA四套模式的2016年6月1至7月31日逐日降水集合预报资料,结合清江流域10个国家基准站观测数据,建立了流域贝叶斯模型平均(BMA)概率预报模型,开展流域多模式集合BMA技术的概率预报试验与评估。结果表明,在清江流域多模式集合的BMA模型最佳滑动训练期长度为40 d,BMA模型预报比原始集合预报有更高预报技巧,比四个原始集合预报MAE平均值减少近11%左右,而对于CRPS除了CMA中心无订正效果外,较其他三个模式平均值提高近15%左右。多模式集合BMA技术能预报降水全概率PDF曲线和大于某个降水量级的概率,同时能给出确定性降水预报,对于极端强降水(大暴雨—特大暴雨量级),BMA 75~90百分位数预报效果较好,对于强降水(暴雨量级),BMA 50~75百分位数预报效果较好,对于一般性降水(小雨—大雨量级),BMA确定性预报结果或50百分位数预报效果较好。
Abstract:Based on the TIGGE datasets from the European Centre for Medium Range Weather Forecasts (ECMWF), the United Kingdom Met Office (UKMO), the China Meteorological Administration (CMA), and the Japan Meteorological Agency (JMA), and its multi-center ensemble systems, and observations in the Qingjiang River Basin, Bayesian model averaging (BMA) probability forecast models were established. The results showed that the optimal length of the training period is about 40 days, and the BMA models for multi-center ensemble outperform those for single center system for lead times of 24 h. The mean absolute error (MAE) and continuous ranked probability score (CRPS) skills of the BMA models are improved approximately 11% and 15%, respectively, compared with those of raw ensemble forecasts. In operation, when the BMA 90 percentile predicted precipitation is extreme precipitation [[50-100) mm·(24 h)-1], the 75-90 percentiles predicted precipitation could be used as the forecast reference, and the heavy precipitation warning could be carried out. For the forecast of severe precipitation [[50-100) mm·(24 h)-1], the forecast result of the 50-75 percentile predicted by BMA can be taken as a reference, while for the general precipitation [≤25 mm·(24 h)-1], the reference of BMA deterministic forecast is relatively strong. BMA probability forecast could give both the PDF curve with full probability and the probability greater than a certain precipitation intensity, which could provide the basis for the probability forecast in operation. However, the small probability value is often ignored, resulting in omission. So how to capture more useful information through the probabilistic prediction method and increase the accuracy of the prediction of extreme weather events will be a challenge for the probabilistic prediction technology.
文章编号: 中图分类号: 文献标志码:
基金项目:国家重点研发计划(2018YFC1508002和2018YFC1507200)、湖北省自然科学基金项目(2018CFB706)、湖北省气象局科技发展基金项目(2018Q02)及中国气象局武汉暴雨研究所基本科研业务费项目(IHRKYYW201815、IHRKYYW201909和IHRKYYW201911)共同资助
引用文本:
祁海霞,彭涛,林春泽,彭婷,吉璐莹,李兰,孟翠丽,2020.清江流域降水的多模式BMA概率预报试验[J].气象,46(1):108-118.
QI Haixia,PENG Tao,LIN Chunze,PENG Ting,JI Luying,LI Lan,MENG Cuili,2020.Probabilistic Forecasting of Precipitation over the Qingjiang River Basin Using BMA Multimodel Ensemble Technique[J].Meteor Mon,46(1):108-118.
祁海霞,彭涛,林春泽,彭婷,吉璐莹,李兰,孟翠丽,2020.清江流域降水的多模式BMA概率预报试验[J].气象,46(1):108-118.
QI Haixia,PENG Tao,LIN Chunze,PENG Ting,JI Luying,LI Lan,MENG Cuili,2020.Probabilistic Forecasting of Precipitation over the Qingjiang River Basin Using BMA Multimodel Ensemble Technique[J].Meteor Mon,46(1):108-118.
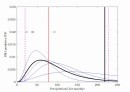